Common Mistakes to Avoid When Hiring Data Scientists
- Sathvik Poojary
- November 21, 2024
- 4 Minute Read
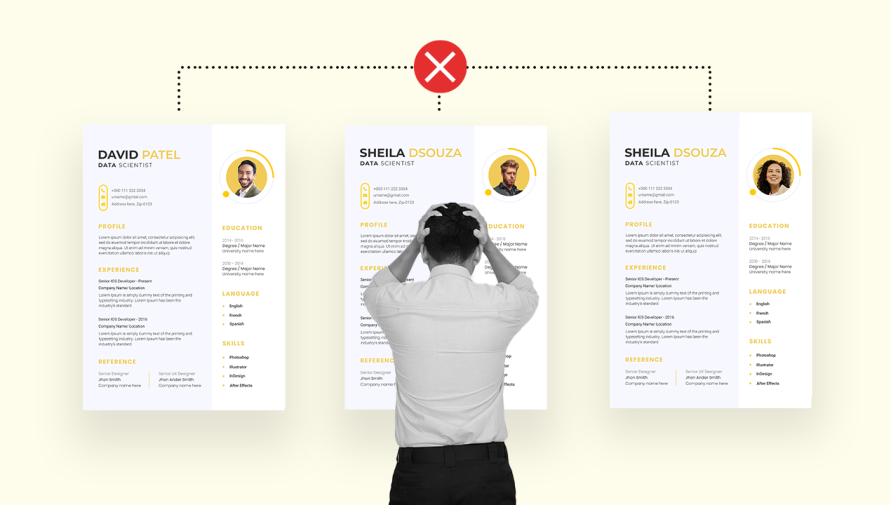
Approximately 80% of businesses worldwide are allocating a significant portion of their profits to the establishment of a proficient data analytics department, therefore employing the most intelligent individuals in the field.
Businesses that wish to stay afloat in this highly competitive market must focus on fine-tuning their hiring strategies.
Whether you are looking to hire data scientists on a full-time or project basis, this guide will help you spot and avoid the most common hiring mistakes. Let’s dive in!
Mistake 1: Focusing Solely on Technical Skills
Hiring data scientists based only on technical expertise is a mistake because it ignores critical abilities like teamwork and problem-solving.
Data scientists frequently collaborate in groups and have to explain their results to stakeholders who are not technical. Even the most technically skilled applicants may find it difficult to deliver business impact and contribute successfully to initiatives if they lack good soft skills.
The tech industry is changing quickly. In a constantly evolving setting data scientists who are flexible and willing to pick up new skills and methods will succeed.
Employers should emphasize a balanced approach that evaluates both technical and interpersonal skills to overcome this.
Mistake 2: Neglecting Cultural Fit
Identifying the perfect match for your organization’s culture is just as important as finding the correct talents when hiring data science professionals.
Ignoring this crucial component will result in a mismatch between your company’s ideals and your new employees. Here’s why it’s important to highlight corporate culture:
- Cultural Fit Is Important: Even if a candidate possesses all the technical abilities you require, an absence of harmony and conflict within your team may result if they fail to align with the business’s values.
- Participation and Drive: Employees who identify with your company’s culture are more likely to be enthusiastic and inspired, benefiting your data science recruitment initiatives and overarching business objectives.
- Retention & Trust: Data scientists will stick with your firm over the long run when they conform to your beliefs.
- Collaborative Setting: Teams frequently collaborate when doing data science. Employing people who share your company’s values promotes a collaborative and peaceful workplace.
Mistake 3: Inadequate Job Descriptions
Inadequate job descriptions while hiring data scientists lead to misaligned expectations and unsuitable candidates.
Why is it important?
- Finding the Correct Applicants: Job descriptions that are too vague may draw a ton of resumes, but they might not be from applicants who possess the abilities you want.
- Burning Funds and Time: Sifting through hundreds of resumes from unsuitable applicants takes a lot of work.
- Misaligned Expectations: When there is an absence of brevity, candidates may have various expectations for the position, which might cause dissatisfaction and even turnover.
A clear description of the necessary skills, such as mastery of programming languages (such as Python and R), familiarity with data visualization tools, and knowledge of machine learning methods, are essential components of a successful job ad. It should also outline educational background and relevant work experience.
By offering a thorough and accurate job description, companies can better match the position’s requirements with their hiring procedure.
Mistake 4: Ignoring Real-World Problem-Solving Abilities
Hiring data scientists without considering their ability to solve real-world problems is a big error, as technical expertise does not ensure successful application in real-world situations.
Data scientists must navigate complicated databases and extract useful insights to solve business difficulties. As a result, practical tests are essential for assessing applicants’ capacity to use their knowledge in authentic situations.
Companies must check how applicants analyze data and convey their conclusions during the candidate evaluation process by creating realistic case studies or challenges. This evaluates technical skills but also exposes critical thinking and flexibility.
Mistake 5: Overlooking Continuous Learning Potential
Since the profession constantly changes with new tools and methodologies, overlooking the continual learning process when hiring it talent like data scientists is detrimental.
Adaptability is essential in data science, where staying current with trends can significantly influence project performance.
Assessing a candidate’s development mindset, or willingness to learn and progress, guarantees competence and adaptability. This emphasis on ongoing education encourages creativity, resulting in a more productive data science team.
Mistake 6: Rushing the Hiring Process
Hiring data scientists too quickly might result in serious errors, such as choosing applicants who don’t have the requisite abilities or cultural fit.
Hasty recruiting frequently leads to the following:
- Omission of important evaluations
- High turnover rates
- Project failures
- Lack of adaptability in employees
To reduce these hazards, organizations should use methods for comprehensive evaluation, such as structured interviews, hands-on tests, and group problem-solving activities.
Moreover, a better fit is ensured by taking the time to evaluate both technical and soft talents.
Mistake 7: Failing to Involve the Right Stakeholders
Integrating technical teams into the hiring process is essential as they offer insights into the particular abilities and resources needed for the position. Hiring choices could ignore crucial technical abilities if they are not consulted.
Additionally, involving stakeholders in the interview process guarantees that applicants share the company’s objectives and ethos. Their viewpoints can be used to evaluate a candidate’s capacity for successfully communicating intricate data findings.
Moreover, including the appropriate stakeholders promotes a better-informed recruiting procedure.
Mistake 8: Not Utilizing Data in the Hiring Process
Not utilizing data in the hiring process for data scientists can lead to subjective decision-making and missed opportunities for finding the best talent.
Hiring managers risk missing applicants with excellent analytical abilities if they lack data-driven insights. To overcome this, companies can use data-driven, organized hiring procedures, such as assessing candidates’ technical proficiency and performance indicators with assessment tools.
Furthermore, examining historical recruiting data can assist in identifying successful characteristics in prior recruits.
Businesses can enhance their hiring results by implementing a data-driven strategy.
Takeaway
Data science is not simply about statistics but individuals who can turn unprocessed data into useful findings.
Hence, achieving success in the global data science workforce market requires striking a balance between academic credentials and practical knowledge, cultural fit, and interpersonal abilities.
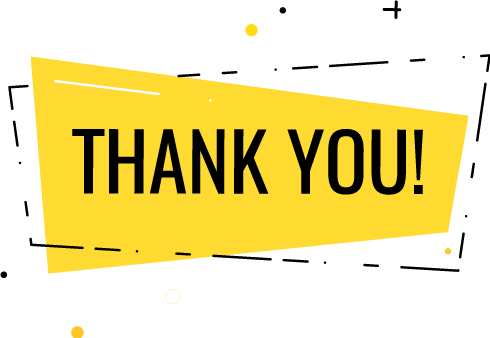
Thank you for submitting the details!
We will keep your information safe. Feel free to contact us with any questions at hello@uplers.com
Please check your email for next steps shared by Robert.